AI in Art Restoration: Reconstructing History with Algorithms
AI is revolutionizing art restoration, from reconstructing lost works to preserving color and detail, bridging past and present in cultural preservation.
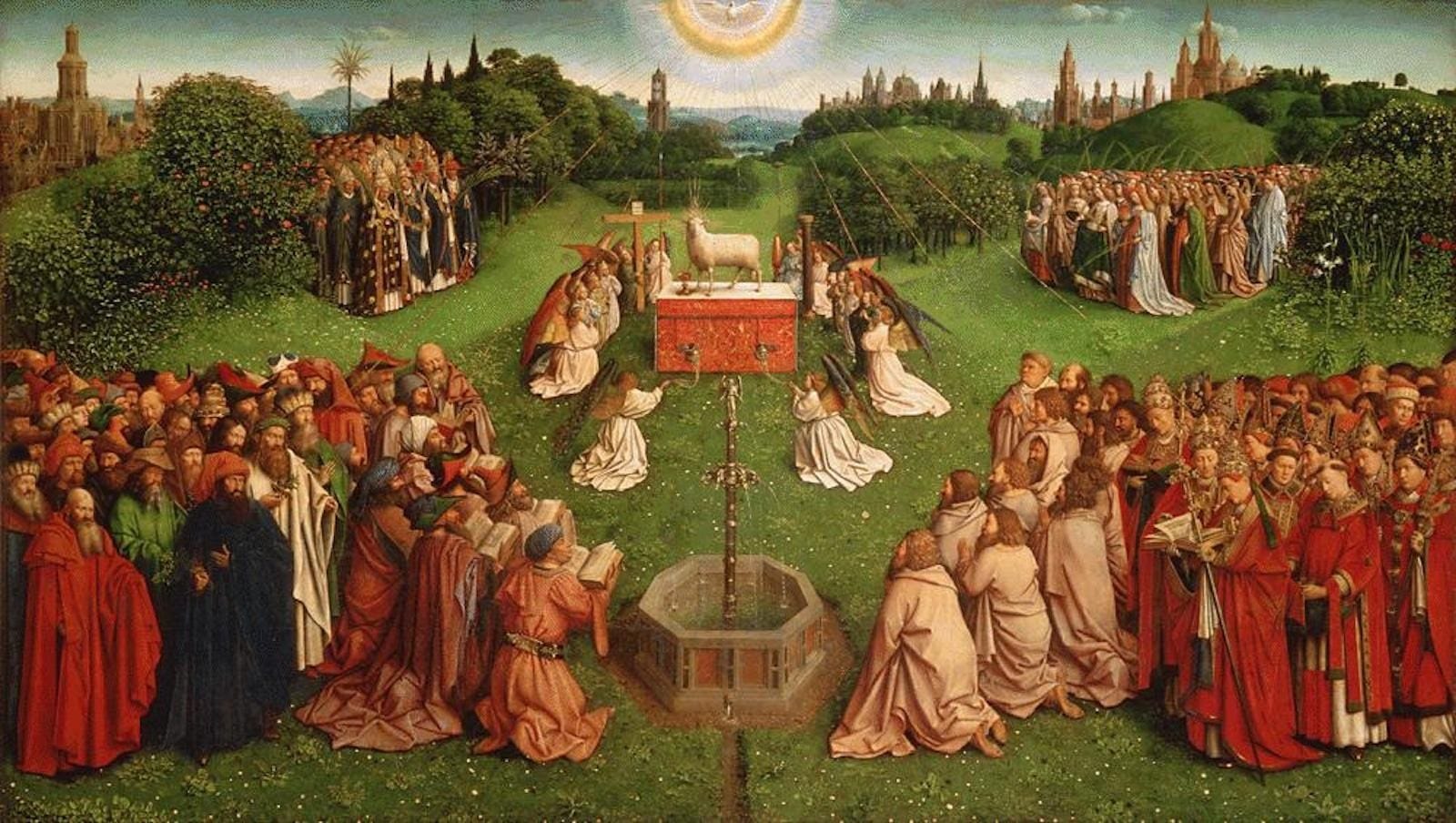
As cultural heritage around the world faces challenges from time, environmental factors, and human impact, art restoration has become a critical field. Recently, artificial intelligence (AI) has emerged as a transformative tool in this space, aiding experts in preserving, restoring, and even reimagining lost aspects of historical artworks. This paper explores how AI is revolutionizing art restoration, examining the methods used, case studies of successful restorations, and the ethical considerations and challenges involved.
Introduction
Art is an invaluable record of human civilization, capturing our cultural, spiritual, and historical narratives across centuries. However, many works of art have suffered degradation over time, whether due to environmental exposure, natural disasters, or human conflict. Traditional art restoration techniques are labor-intensive and often rely heavily on the subjective interpretation of experts, which can sometimes lead to unintentional alterations.
Artificial intelligence presents an innovative solution to these limitations, bringing both efficiency and new possibilities to the art restoration field. By leveraging deep learning and image recognition technologies, AI can analyze and reconstruct artwork with a level of detail that was previously unattainable. AI can even be trained to predict and fill in missing portions of artworks by studying patterns in surviving pieces, enabling restorers to recreate sections that may have been irreparably lost.
Methods and Technologies in AI-Driven Art Restoration
- Deep Learning and Image Recognition
AI models trained on extensive datasets of historical art styles can identify patterns and textures specific to an artist or period. For instance, convolutional neural networks (CNNs) enable AI to analyze brushstrokes and color schemes, guiding restorers in accurately reconstructing missing or damaged parts. By using reference images, these models help restore faded sections while preserving the integrity of the original style. - Generative Adversarial Networks (GANs)
GANs are used to generate new sections for artworks that have missing or damaged areas. Through this approach, a GAN model can learn from complete artworks in similar styles and generate visually cohesive content to fill in gaps, matching the historical context of the piece. - 3D Scanning and Reconstruction
For sculptures and other three-dimensional works, AI combined with 3D scanning creates digital replicas, which can be used to assess and restore the structure. Algorithms can analyze cracks and wear patterns, and even suggest reconstruction approaches that maintain the piece’s authenticity.
Case Studies in AI-Driven Art Restoration
The Ghent Altarpiece (Belgium)
In restoring Jan van Eyck's Ghent Altarpiece, AI-supported high-resolution imaging and X-ray fluorescence were used to distinguish original brushstrokes from overpainting, revealing the piece’s original colors and details.
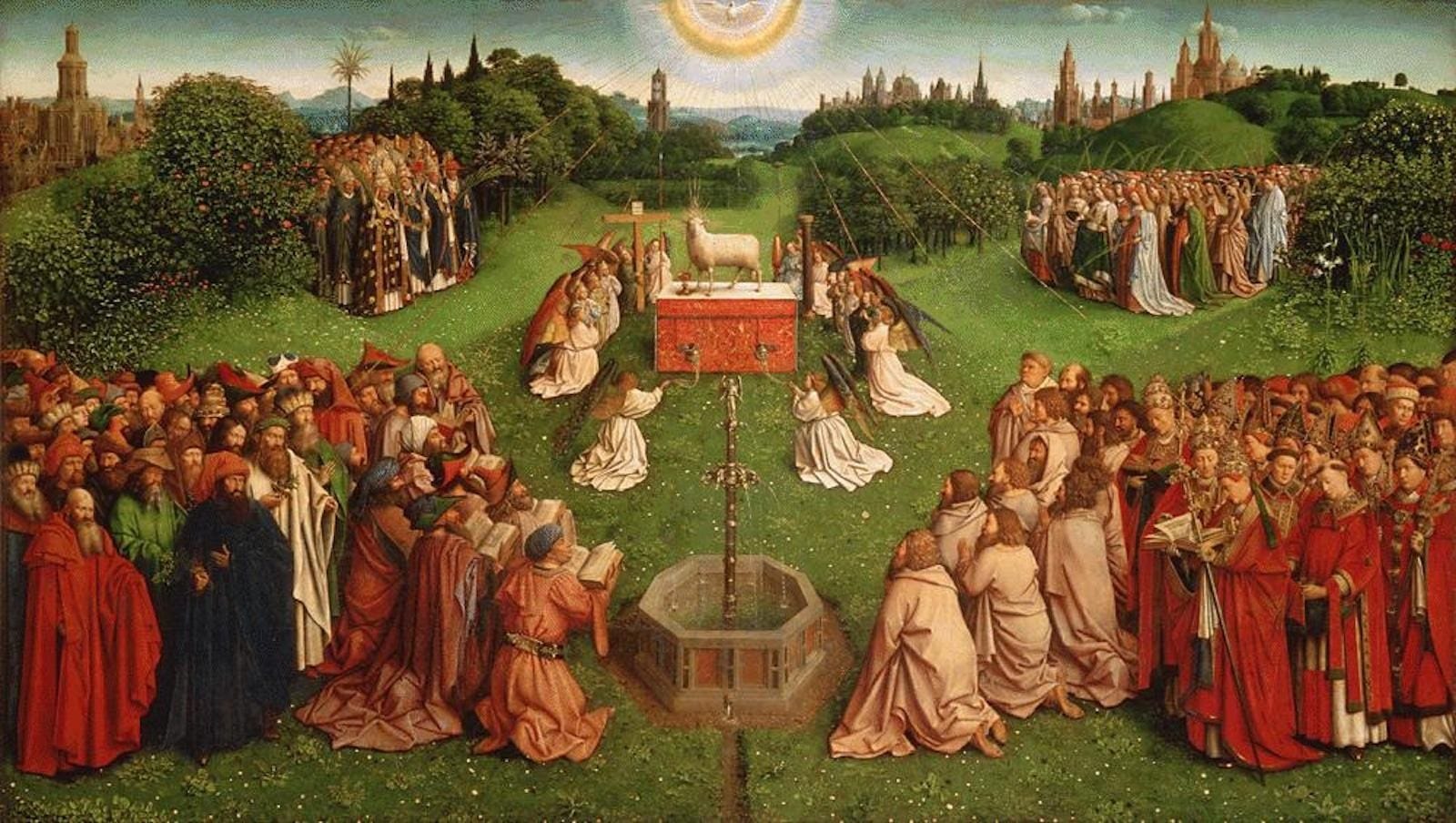
Velázquez’s Expulsion of the Moriscos (Spain)
In March 2024, artist Fernando Sánchez Castillo utilized AI to recreate Diego Velázquez's lost painting, Expulsion of the Moriscos, which was destroyed in a 1734 fire. By analyzing historical descriptions and preparatory sketches, AI generated a video representation of the artwork, offering insights into its original appearance.
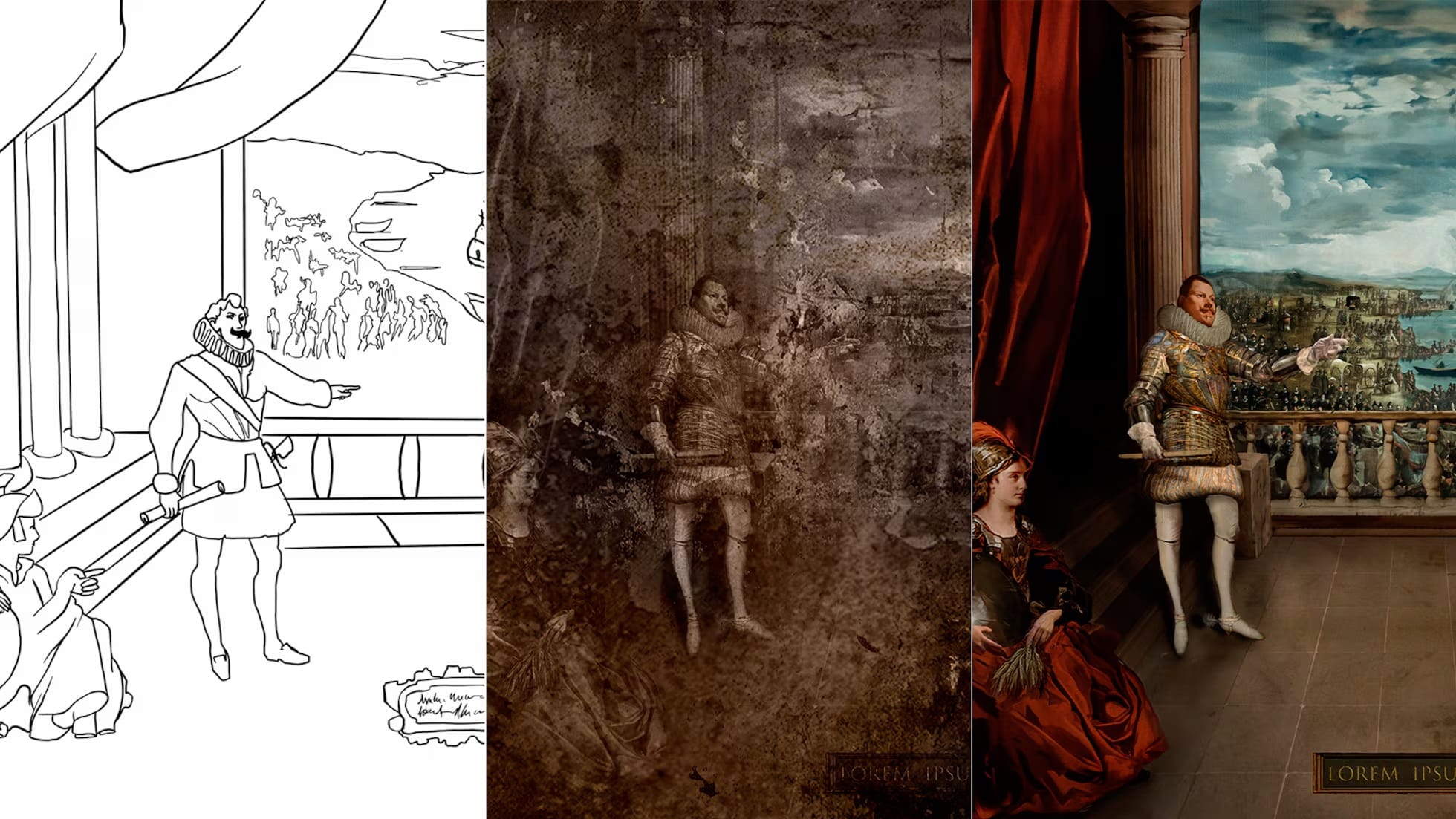
Pompeii Archaeological Site (Italy)
AI-driven image analysis at Pompeii monitors and detects new cracks or fading in frescoes, comparing current images with historical records to guide preventive conservation efforts.
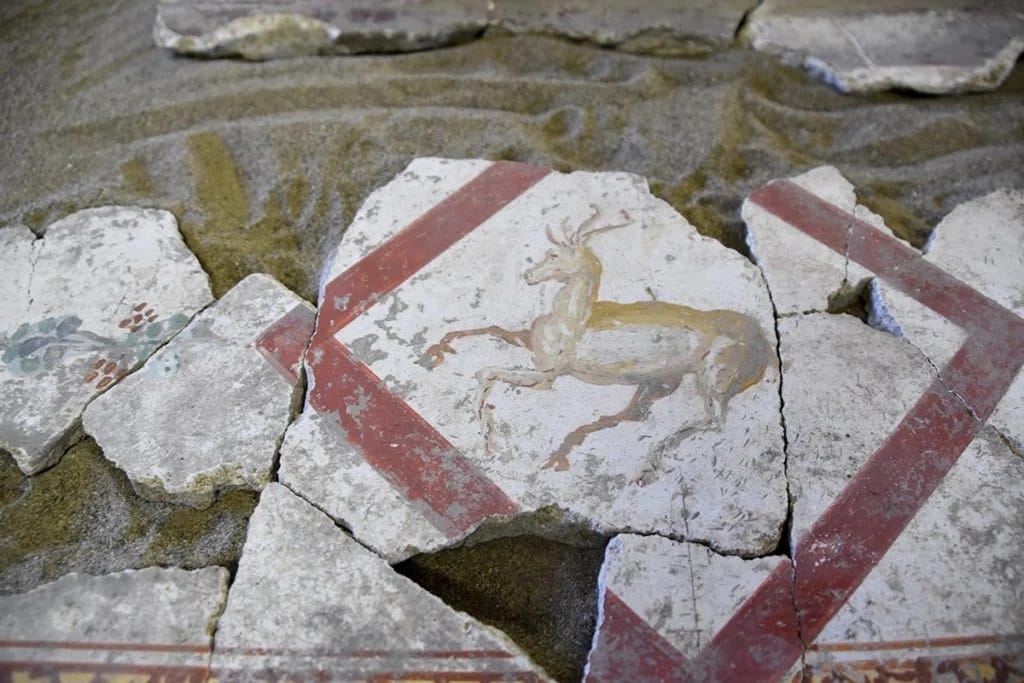
Notre-Dame Cathedral (France)
Following the 2019 fire, AI-based 3D modeling helped recreate Notre-Dame’s architecture, analyzing past scans to guide restoration methods that align with the cathedral’s Gothic style.
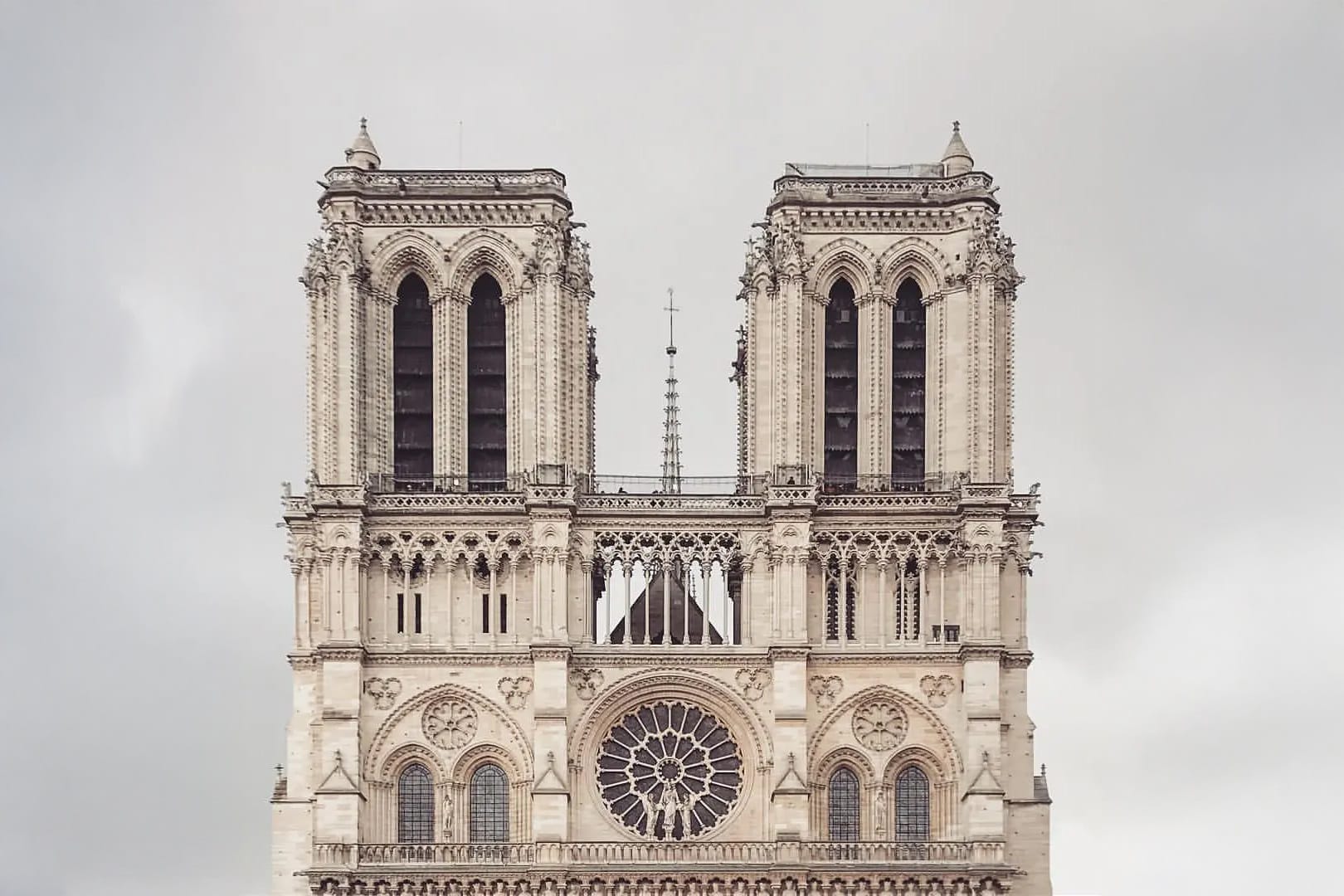
Beyond the Data: Navigating AI’s Limits in Art Restoration
AI’s potential in restoration is vast, but it’s also constrained by the need for high-quality, extensive datasets, something that’s often lacking for ancient or unique art. Training AI on rare styles or one-of-a-kind techniques is a significant hurdle, as models rely on patterns from existing data. This limitation raises a fascinating question: can we teach AI to recreate what’s never been fully documented? Moving forward, hybrid approaches that blend AI’s analytical power with human creativity could redefine restoration, allowing technology to set the foundation while experts bring a personal touch, ultimately balancing innovation with historical integrity.